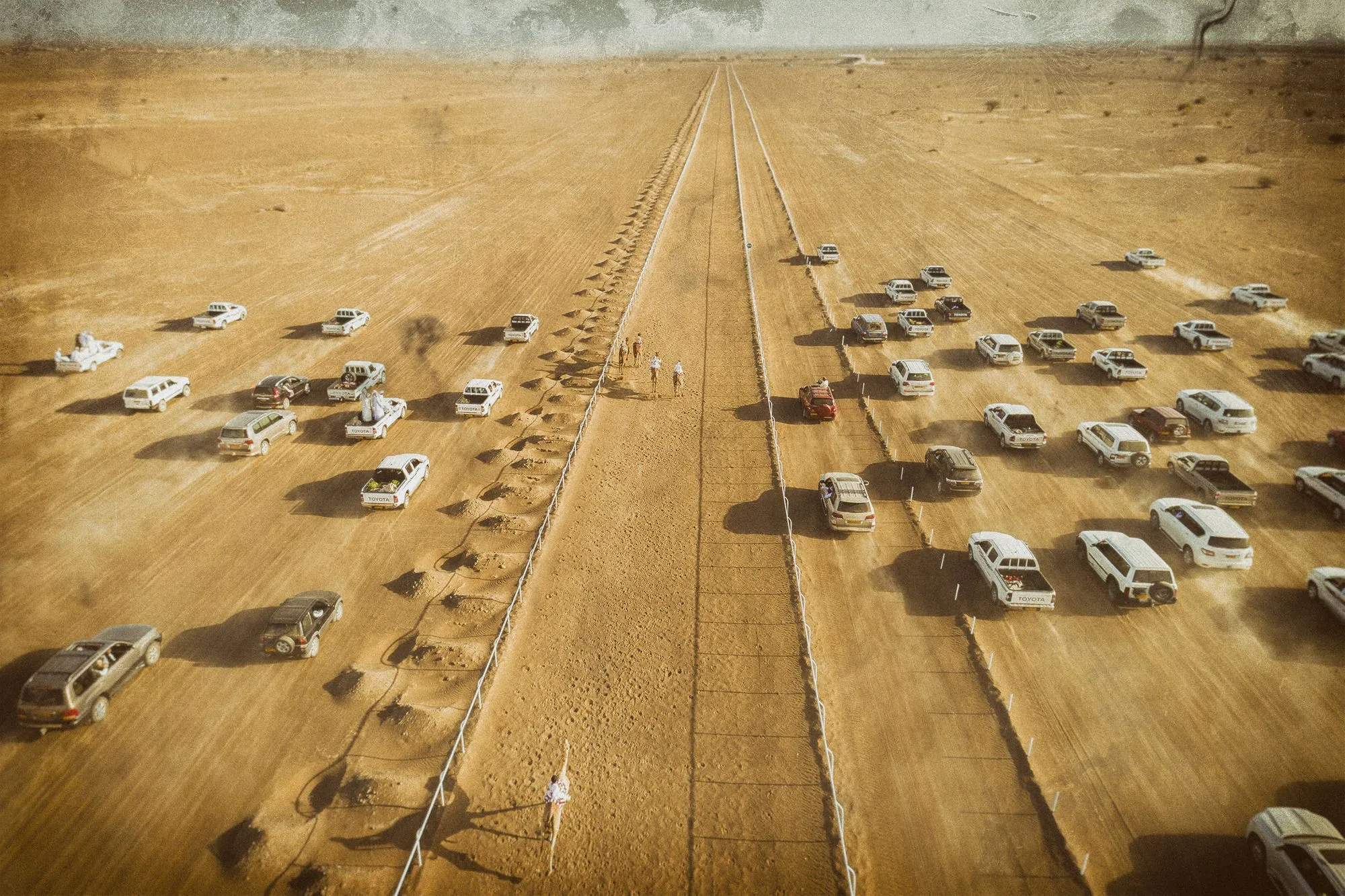
Top business drivers of real-time analytics and machine learning in retail
October 31, 2017 • 6 min read
The retail industry is embracing big data, analytics, and machine learning (ML) to improve customer engagement, optimize operations, and drive sales. Increasingly, we’re seeing business intelligence systems that were once based on historic data, offline modeling, and traditional reporting being replaced by algorithms that operate on real-time data about customers and the world at large. They can respond to real-time events to generate personalized offers, compute dynamic pricing, and optimize order sourcing locations in real-time. In this blog post, we look at the business drivers of real-time applications in retail and outline the top IT initiatives responsible for the increasing budget allocations for the implementation of real-time capabilities in 2017 and 2018.
To get a better sense of how real-time processing is shaping digital retail, let’s start by getting a better sense of what “real-time” means. Consider the steps that happen between a business event and an automated response:
In the past, traditional business intelligence (BI) tools reported on trends and offered sales projections from data about events that was weeks or months old. That’s the equivalent of figuring out how to drive by looking at the rear-view mirror. The industry is now getting to the point where data lakes gather information within a 24-hour window via extract-transform-load (ETL) processes and allow organizations to run nightly models and come up with business insights that can be deployed to operational systems the next day.
As a next frontier, new real-time streaming systems are allowing businesses to act on new insight within seconds, providing opportunities for applications that personalize user experience, compute dynamic pricing, or make intelligent sourcing decisions in true real-time.
Think of the value of real-time systems in terms of three different operations, as illustrated in the figure below:
- How long does it take to respond to a specific event given a complete model?
- How long does it take to run the model?
- How long does it take to “learn” and adopt the model?
The value of these operations increases as we add real-time capabilities to each level as we move down the list.
To get even better sense of how this pyramid of real-time value works in practice, let’s consider personalization. At one time, the best attempt businesses could make at personalizing coupons was publishing them in a newspaper that served a particular geographic area, or sending them out in a direct mail campaign to a particular postal zone. Today, retailers can customize their offerings based on historical and demographic data they’ve gathered on groups of customers — sex and age information, income levels, and so on. This is a fairly simple way to use the most easily acquired data. All target customers with the same characteristics — all 18-year-old women in ZIP code 90210, for instance — will get the same coupon.
Note that this level of interaction requires no real-time data. Think of it as the base level of a personalization pyramid (see figure) where the value of personalization rises as we incorporate greater levels of real-time information. While this level of customization used to be all that retailers could manager, it’s not enough to provide businesses with a competitive advantage.
Businesses can do a lot better moving from segmentation-based personalization to individualized offers by incorporating individual history, profile data, and preferences, in addition to the segmentation — providing a coupon for someone to purchase an item they’re interested in on their birthday, for instance. This requires a 360-degree view of customer data — personal profiles, order and shopping history, even click streams. Getting that 360-degree view depends on data collection and aggregation from multiple systems to create a data lake. The personalization models we can derive from such a data lake are far more targeted than segmentation-based personalization and lead to significantly higher conversion and better margins.
A still higher level of personalization requires real-time situational context — a glimpse of where a customer is or what she might want to buy at a given moment. With real-time information, retailers can make offers that appeal to customers right at the register — giving them a deal on a bundle of goods when they come to purchase a few individual items, or a discount for purchasing multiple items. Deals like these don’t just lead to not higher conversion rates than those involving non-real-time data, but can also optimize margins and inventories.
There’s an even higher level of sophistication to which real-time data can take personalization. If a business can keep a real-time eye on more than just its customers, but also its inventory, supply chain, and even real-world events like weather patterns, it can exploit that data to offer precisely targeted deals – offering to deliver an umbrella to a customer’s office on a day when an unexpected thundershower pops up, for instance. To exploit that opportunity, businesses need real-time insight into product availability, competitive pricing, and demand surges. Systems to provide that information are the most complex of all— but also the most valuable.
Top business drivers of real-time applications in retail
In the last 12 months we’ve seen six areas where investment in real-time applications promises massive improvements in conversion rates and overall profitability. We’re working with many retailers on implementing new systems in these areas, and expect to see more budgets allocated for real-time applications in 2018. Let’s take a quick look at each of the areas:
Personalized search: Search may be the hottest topic in personalization nowadays. Until recently, when a customer searched for “little black dress,” it was difficult to customize search results depending on whether the customer was an 18-year-old who shops at the local mall or a 40-year-old who only buys designer labels. Now, if we can incorporate more knowledge of the purchaser, we can tailor the results to provide better choices and enhance the likelihood of making a sale.
Personalized offers: This is the example we talked about earlier. Retailers can motivate immediate purchases more successfully by offering deals based on what they know about their customers’ history and their current context.
Dynamic pricing: The ideal price for an item depends on its availability, market demand, the competition’s price for the same item, and even a customer’s individual context and willingness to purchase. Setting that ideal price would be the Holy Grail for businesses using real-time data to maximize their opportunities.
Dynamic inventory: How much of each item should a business keep in stock or on the shelves at each of its stores? Retailers don’t want to be stuck with items that won’t sell and therefore have to be discounted, or fail to stock items that are selling quickly. Of course businesses may keep safety stock on hand to mitigate the risk of inventory shortfalls, but trendy items can become quick sellers faster than suppliers can ship them. Systems that track real-time fluctuations in inventory and demand can help maximize sales and revenue.
Intelligent sourcing: Large retail chains have lots of stores and a smaller number of distribution centers, plus online shopping applications. Nowadays, many retailers are treating their stores as distribution centers — forwarding orders to local stores and shipping items from there. The level of intelligence you apply to this process can have a big effect business margins. Shipment costs, inventory considerations, service-level promises, and manpower issues all factor into the issue, and optimizing the solution is a complex problem. Machine learning based on real-time data can help businesses plug any margin drain.
Real-time alerts: Anytime an unusual pattern pops up, or you find some of your data outside of the range you expect, you should get an alert. For instance, if your inventory is running low in a particular store, or you don’t have enough manpower on a particular day, you should find out about it as soon as possible. The object is to avoid losing out on revenue opportunities.
Conclusion:
Our clients are now taking advantage of real-time data in these areas to optimize their revenues and provide new opportunities for their customers. From these initiatives we’re seeing new levels of personal targeting in e-commerce platforms and loyalty programs, as well as new systems that adapt to inventory and supply chain conditions more quickly than the systems our clients ran just a few years ago.
We’ve been helping retailers implement real-time applications and accelerate their time to market. Learning how to capture, correlate, and process that data can take a significant investment in both money and time, and developing systems to leverage real-time data is a complex and often expensive proposition, but we’ve found that building and deploying personalized, real-time retail applications can provide a beneficial bump to a business’s bottom line.