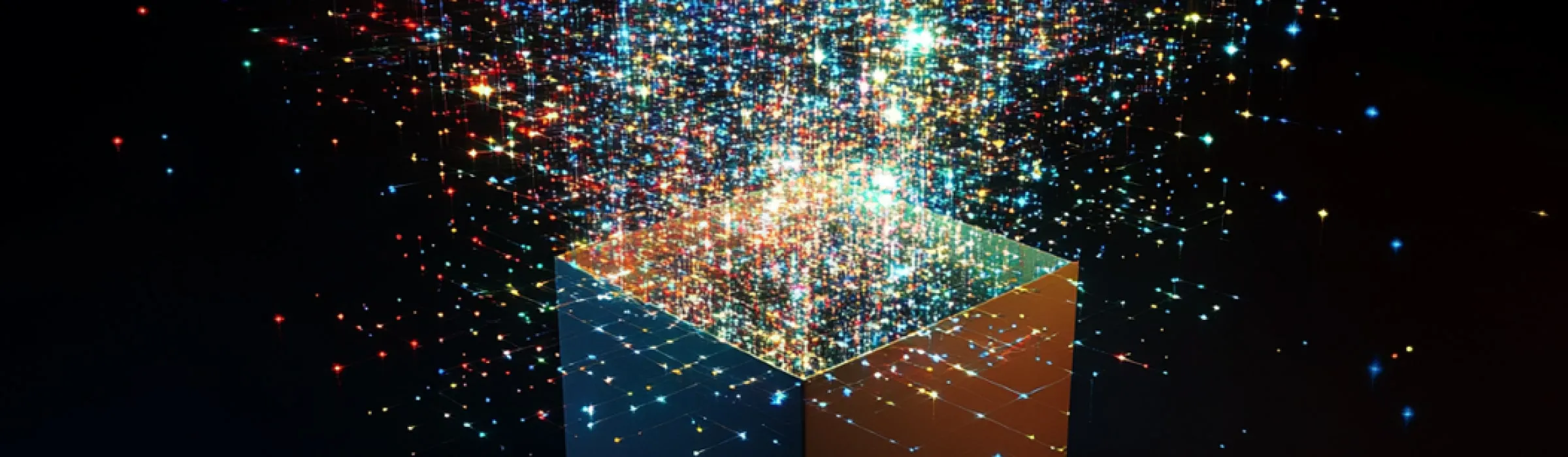
Data as a product: The missing link in your AI-readiness strategy
April 8, 2025 • 7 min read
Most enterprise leaders dip their toe into AI, only to realize their data isn’t ready—whether that means insufficient data, legacy data formats, lack of data accessibility, or poorly performing data infrastructure. In fact, Gartner predicts that through 2026, organizations will abandon 60% of AI projects unsupported by AI-ready data.
But what’s the link between data quality and AI outcomes?
At its core, AI is the process of taking data and using it to predict outcomes, generate information, or perform actions. However, until now, enterprise data has typically been limited to “information humans need to make a decision.” To hand off some—or all—of that decision-making to AI, the contextual information we have through experiencing the world, must be distilled into “data.”
Recent advancements in AI have thus been a wake-up call for companies that underinvested in data management and strategy to date.
As AI initiatives scale across the enterprise value chain, data teams are feeling the pressure. Requests to “make data available for AI” are piling up—but many organizations are still carrying a hangover from the big data era: massive volumes of unused and no longer useful information. That’s because modern AI demands outcome-oriented data that enables prescriptive, actionable insights—very different from the descriptive or predictive models of the past.
This growing urgency around data modernization—a top AI trend for 2025—is reshaping how organizations think about and manage data—driving the adoption of new tools, techniques, and mindsets that support more mature, AI-ready practices.
To move forward, data leaders must establish a modular data architecture, embrace data-as-a-product (DaaP) principles, and prioritize governance so that data can fuel both operational excellence and new revenue opportunities. It all begins with understanding the data you have, the data you need, and the gap in between.
In this blog, we’ll explore what that data modernization journey looks like.
The gap between legacy and AI-ready enterprise data
Today, every enterprise is on the path to AI maturity with the goal of driving efficiency, efficacy, and ultimately transformation:
- Efficiency: The enterprise is ‘exploring AI’, looking to use it as a tool for task efficiency.
- Efficacy: The enterprise has built up AI skills and data readiness and is now looking to improve the outcome of processes.
- Transformation: The enterprise is fully AI-enabled and uses skills and tools alongside internal and external data for new business opportunities.
The data function must stay ahead, proactively preparing data to enable organizations to move seamlessly to the next maturity stage. This translates into enabling modern data techniques for each stage.
But getting to this stage isn’t as straightforward as it sounds. There is a huge gap between legacy data and AI-ready data.
Ten years ago, data teams typically began by identifying key data sources and understanding how that data was being reported. As they moved up the maturity curve, the focus shifted to introducing business intelligence (BI) and descriptive analytics, followed by centralizing data to unlock diagnostic insights. From there, predictive analytics became a priority—especially in sales, marketing, and personalization. External data usage was minimal and often limited to benchmarking, while dedicated data leadership was seen as a marker of only the most advanced, transformational organizations.
Today, the pace is very different.
Modern data journeys begin with cloud-based platforms, basic data governance frameworks, and self-service analytics tools. From there, enterprises quickly enable organization-wide analytics and real-time automated reporting. The next step is using machine learning and AI to optimize operations, deliver Data as a Service (DaaS), enable real-time data streaming, and apply natural language processing (NLP) to extract value from unstructured data.
As maturity grows, organizations begin building monetizable data-driven insights, and AI becomes more than a tool—it starts acting as a co-worker. This includes Agentic AI-powered functions and experiences, such as customer service chatbots. The ultimate goal is to evolve into a fully AI-driven organization, where data and intelligence are embedded across every function.
How adopting a data-as-a-product (DaaP) mindset fuels AI maturity
To support AI maturity, businesses must modernize how data is owned, managed, and delivered. This requires shifting the organizational mindset to treat data as a product by empowering business teams to take ownership of data collection and quality, while expert data teams support building the right skills, tools, and governance to ensure the data is delivering value and AI success.
Redefine data practices to promote domain-oriented data ownership
Historically, data teams were the primary owners of enterprise data. But thanks to frameworks like data mesh, that model has changed. Business units now take ownership of their own data—responsible for collection, quality, governance, and ongoing data stewardship—while data teams shift into an enabling and governance role.
This support needs to start early, well before AI is introduced. Much of the foundational work is now commoditized: helping teams build pipelines, structure datasets, and implement basic governance practices.
The real value comes when the data team steps into its evolved role, enabling business domains to unlock the full potential of their data. Modern data functions should be:
- Owning data governance and observability strategies
- Helping teams unlock data value
- Connecting departments to enable smarter, cross-functional data use
- Ensuring data is structured and contextualized for AI readiness
- Bridging skills gaps through education and enablement
- Contributing directly to AI product development (For example, prompt engineering, model tuning)
In this new structure, data teams act as the glue between business functions and development teams, empowering business functions while collaborating with platform teams to drive AI success.
Embrace a data-as-a-product mindset
As AI usage matures, companies are rethinking how data is managed—treating it like a product with clear ownership, defined consumers, and measurable outcomes. This shift toward a data-as-a-product mindset ensures data is not only trusted and usable but also purpose-built to support AI initiatives.
To successfully implement this mindset, data must follow four product-design principles:
- Anticipate: Understand and proactively meet the needs of data consumers
- Advise: Provide insights that support evidence-based decision-making
- Adapt: Serve different users with tailored access to relevant data
- Act: Enable users or systems to take actions based on the data provided
A true data product should embody the following characteristics:
- Discoverable: Users can easily search and explore relevant datasets.
- Addressable: Programmatic access remains stable despite schema or access pattern changes.
- Understandable: Well-documented to reduce tribal knowledge and improve literacy.
- Trustworthy and truthful: Meets SLOs for timeliness, lineage, accuracy, completeness, precision, and freshness.
- Natively accessible: Usable within the tools and environments familiar to end users.
- Interoperable and composable: Follows standards for data integration and reuse.
- Valuable on its own: Purpose-driven with transparent cost and resource usage.
- Secure: Enforced access control, encryption, data retention, confidentiality, and regulatory compliance.
Here, data can be called using traditional ETL or modern techniques such as RAG pipelines.
To get to data products quickly from existing data sets, the Data Build Tool (DBT) is gaining popularity. It is a data modernization technique that enables organizations to transform raw, unstructured data from data lakes into structured, consumable data products using SQL-first methods and software engineering best practices—without needing to build new pipelines from scratch.
Build an AI-era data team
As enterprises redefine their data practices and adopt a data-as-a-product mindset, the skills and composition of the AI-era data team must evolve in parallel. Roles in data engineering, data science, and ML/AI engineering now require significant upskilling, particularly in areas like data migration, guardrails, observability, cost optimization, and end-to-end support.
For example, modern ML engineers must develop deep expertise in LLM infrastructure, retrieval-augmented generation (RAG), and continual learning to build scalable AI applications.
This upskilling must go hand in hand with automation to reduce manual efforts, enhance efficiency, and accelerate AI adoption. AI-driven tools can help optimize workflows, and implementing structured processes can support ongoing data modernization while minimizing operational bottlenecks.
Establish advanced DataOps practices for continued success
As data practices and operating models evolve, traditional data operations simply can’t keep up. Advanced DataOps techniques are critical to the success of data modernization efforts and managing modern data complexity.
Enterprises can standardize processes to efficiently ingest and process both structured and unstructured data, implement robust frameworks for prompt engineering and vectorization to optimize unstructured data management, and enforce guardrails and semantic caching to ensure data integrity and reliability.
These practices help organizations adapt to evolving data formats and use cases, aligning storage and retrieval strategies with business needs. However, no single tool fits all purposes—which is why we help businesses establish customized DataOps processes and best practices to maintain long-term efficiency, scalability, and AI readiness.
Can AI help power DataOps?
Yes, AI can help modernize the very data it will later consume.
Enterprises must leverage AI to improve data quality beyond just automating checks for null or empty values. It must be integrated across the entire DataOps pipeline—including exposing quality metrics to data catalogs—to enable unsupervised anomaly detection, root cause analysis, and AIOps. Dashboards to track all quality checks help teams validate data consistency and quickly resolve issues before they impact downstream processes.
The AI-driven DataOps framework comprises several interconnected components:
- Catalog and metadata management services: AI helps manage metadata with robust schema management and full-text search, maintains a glossary-based knowledge base, provides lineage tracking, and seamlessly integrates with quality checks and pipelines.
- Data quality services: AI generates automated quality checks, deploys anomaly detection algorithms, and integrates tightly with existing data pipelines and metadata systems, providing continuous, proactive data integrity.
- Data pipeline orchestration: Complex, interdependent data workflows become scalable through AI-powered orchestration, which handles hundreds of flows and proactively alerts teams of issues.
- Access control: Ensuring compliance with privacy regulations such as GDPR and CCPA, AI supports rigorous dataset- and field-level security through encryption and access governance.
Conclusion
As every enterprise aims to capitalize on AI, data remains the foundation for success. The demands on enterprise data teams have shifted, and even previously mature organizations now find themselves at the beginning of a new data maturity ladder. There’s no avoiding the work required to modernize data estates—but AI can certainly accelerate delivery and improve operations in this new world. Furthermore, by rethinking data practices, embracing a data-as-a-product mindset, and equipping teams with the right skills and tools, businesses can unlock AI’s full potential.
At Grid Dynamics, our data experts have conducted numerous enterprise-wide assessments to identify data modernization needs, helping organizations succeed in their Data and AI initiatives. Contact us today to schedule your Data and AI readiness assessment.
Frequently asked questions
What is data as a product (DaaP)?
Data as a Product (DaaP) is an approach in data management where data sets are treated as standalone products, designed, built, and maintained with end users in mind by applying product management principles to the data lifecycle, with a focus on quality, usability, and user satisfaction.
What are the foundations of DaaP?
DaaP is built on domain-oriented architecture, product thinking, and self-service infrastructure, requiring data to be discoverable, addressable, trustworthy, well-documented, interoperable, and secure to ensure reliable and AI-ready usage.
What’s the difference between DaaP and data products?
DaaP is a data management methodology that packages data, code, metadata, and infrastructure as a reusable asset for enterprise-wide access, while data products focus on leveraging this data to deliver actionable insights and solutions, such as analytics dashboards and predictive models, to solve defined business problems.
How can teams implement data as a product?
Teams implement DaaP by assigning domain ownership, adopting product management principles for data, and modernizing pipelines. Tools like DBT help accelerate this process by transforming raw, unstructured data from data lakes into structured, consumable data products using SQL-first methods and software engineering best practices—without the need to rebuild pipelines from scratch.
What does DaaP mean for the enterprise?
DaaP encourages enterprises to treat data as valuable products by applying product principles to its management, selection, customization, and delivery. This approach focuses on collecting only purposeful data, organizing it clearly, making it user-friendly, and ensuring it aligns with industry and domain needs—enabling more effective, trusted, and impactful use of data across the organization.
What challenges does DaaP present?
Adopting DaaP brings challenges such as navigating complex data privacy regulations, mitigating security risks, and overcoming organizational resistance to change. Successful implementation requires robust security, clear governance, and effective change management. Cultural shifts and data literacy gaps also pose hurdles, as employees must adapt to new ownership models and data practices forcing organizations to invest in training, transparent data practices, and user-friendly tools to empower teams and fully realize DaaP’s potential.