Get the White Paper
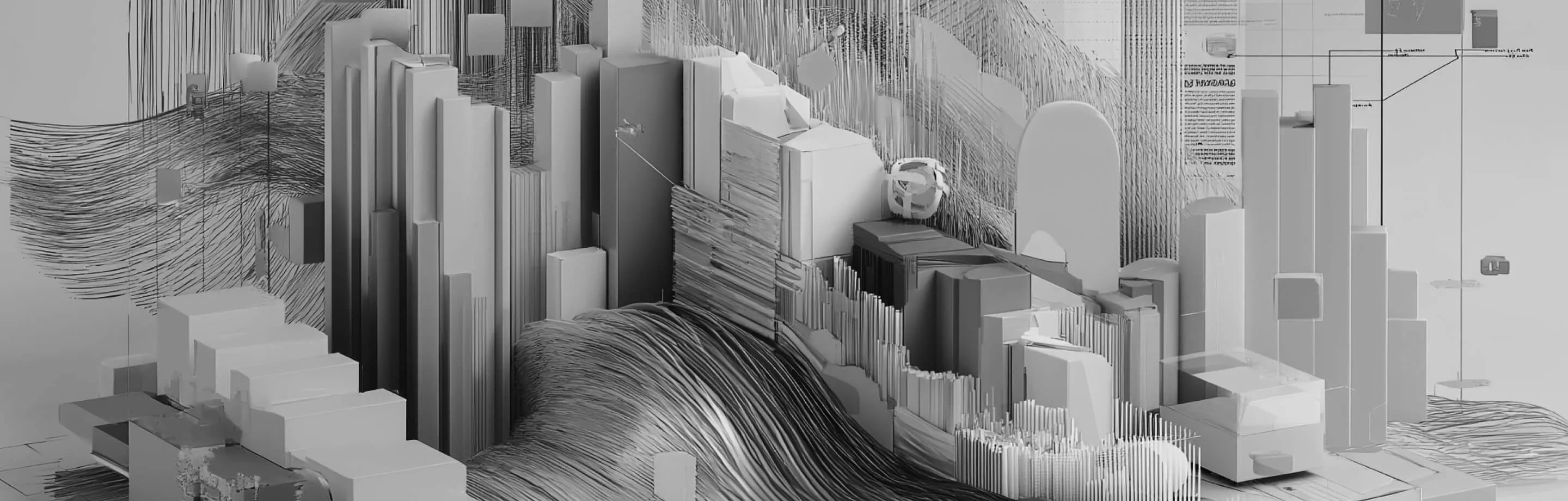
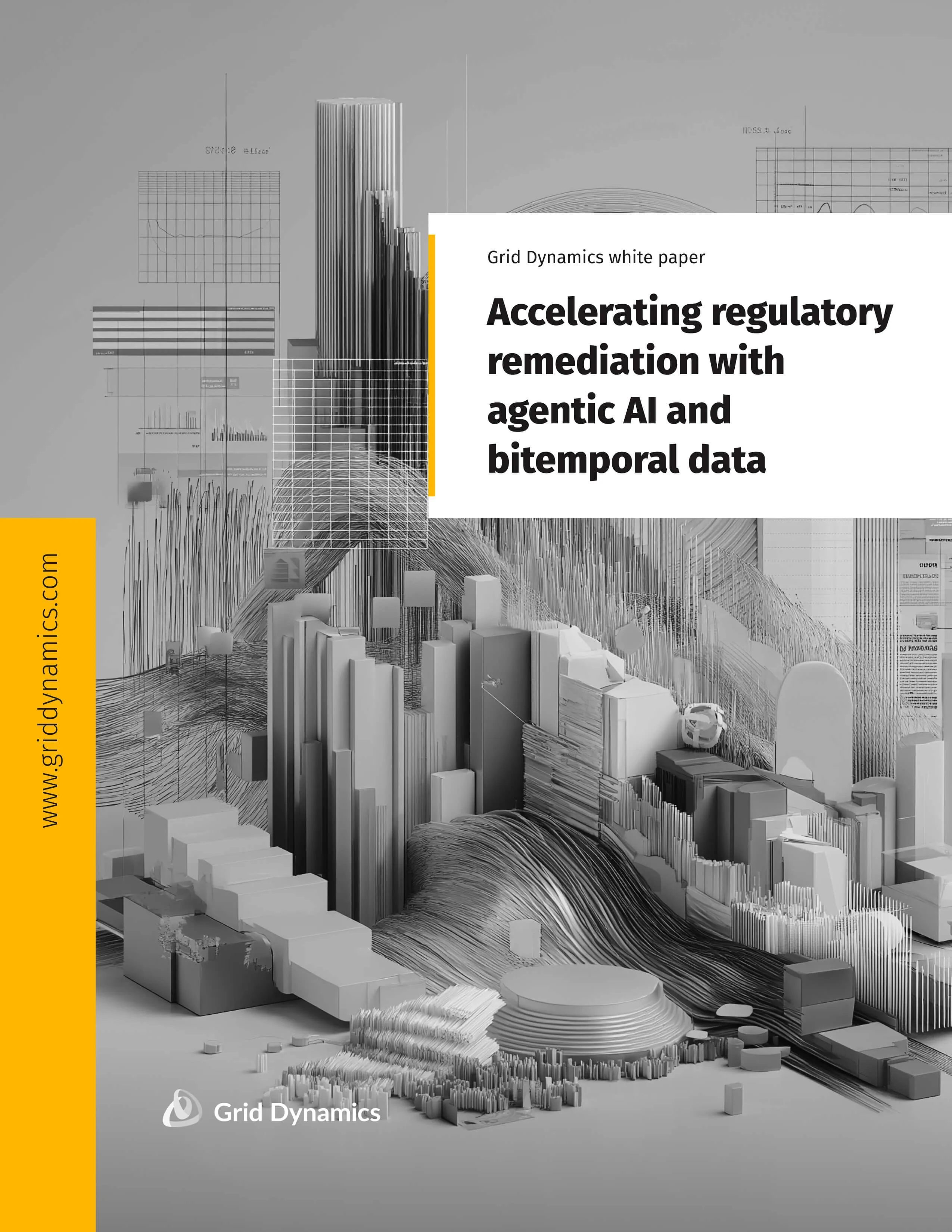
Accelerating regulatory remediation with agentic AI and bitemporal data
Wealth managers, asset managers, and banks face mounting pressure when it comes to regulatory remediation—the process of responding quickly and accurately to inquiries from regulators like FINRA and the SEC. These demand answers to complex questions about past transactions, communications, and decisions within days or hours. Yet many organizations still rely on fragmented data and manual processes, making it difficult to reconstruct historical records and respond with confidence.
This whitepaper explores these compliance challenges and introduces a modern, integrated platform that combines Agentic AI regulatory compliance tools with bitemporal database technology (XTDB). Instead of scrambling to piece together historical data, AI agents translate natural language inquiries into precise data queries against an immutable, time-aware database.
Firms that adopt this regulatory remediation solution can expect to deliver faster regulatory responses (often within 24 hours), a 30% or more reduction in manual effort, and enhanced confidence in their compliance posture.
As a leader in digital transformation and the creator of the open-source XTDB database—with a track record of over eight years of AI engineering expertise, Grid Dynamics plays a key role in delivering this next-generation compliance solution.
Download the full white paper now to learn how our agentic AI and bitemporal data-powered solution can accelerate your regulatory responses, ensure accuracy with comprehensive audit trails, and reduce operational burdens.
Regulatory compliance challenges
Regulators like FINRA, SEC, FDIC, and the Federal Reserve often issue inquiries such as “Who knew what and when?” or request organizations to reproduce records exactly as they were at a specific point in time.
However, meeting these demands is difficult due to key challenges:
- Fragmented & siloed data: Data is scattered across systems with inconsistent schemas and identifiers. Investigations often require collecting data from multiple disconnected sources with no unified view—slowing response times and increasing the risk of errors.
- Manual, ad hoc workflows: Analysts rely on SQL, Python scripts, spreadsheets, and emails. Operational staff may lack the required expertise, forcing them to depend on IT teams for data pulls. Ad hoc processes are difficult to audit or standardize, increasing operational risk.
- Evolving metadata and relationships: Key reference data—such as account ownership, instrument identifiers, and organizational hierarchies—changes over time. Legacy systems often don’t retain prior states, making it difficult to reconstruct historical context.
- Lack of historical reconstruction: Conventional databases focus on the current state. Prior values are either overwritten or archived in logs that are difficult to query, making it challenging to answer, “What did we know on X date?”
- Time pressure and accuracy: Regulators demand accurate responses within 24–48 hours. Firms must collect, validate, and format data to meet regulatory standards. Missing deadlines or submitting incorrect data can result in fines, sanctions, and reputational damage.
How bitemporal data and agentic AI help tackle regulatory compliance challenges
Unlike traditional databases, XTDB—an open-source bitemporal database developed by Grid Dynamics—is purpose-built to retain a complete history of data. XTDB tracks both valid time (when the data was true in the business context) and transaction time (when the data was recorded).
While XTDB provides historical data and time-aware query capabilities, agentic AI intelligently leverages this data. Through autonomous reasoning and action cycles, it interprets regulatory inquiries posed in natural language and generates precise responses by querying the underlying database.
Key benefits of a bitemporal database for compliance
XTDB delivers specific advantages to effectively manage compliance requirements:
- Immutable, dual timeline data store: XTDB maintains both valid time and system time, never overwriting records. This allows firms to retrieve accurate historical states—crucial for audits and inquiries like “What did you know and when?”
- Reconstructing historical states on demand: With all versions retained, compliance teams can easily recreate past views of data to meet requirements like FINRA Rules 4511 and 3110 using standard SQL queries.
- Regulatory auditability and data integrity: Every change is timestamped and preserved, enabling tamper-proof audit trails and reducing risk during disputes or investigations.
- Seamless integration & schema flexibility: XTDB supports schemaless ingestion, making it ideal for fragmented systems. It offers PostgreSQL compatibility and XTQL for flexible querying across evolving data models.
- Open-source and enterprise-ready: Led by Grid Dynamics through JUXT, XTDB is cloud-native, scalable, and integrates with modern data tools. It offers on-prem or cloud deployment without licensing complexity.
How agentic AI effectively utilizes historical data
Agentic AI, one of 2025’s most trending AI technologies, provides the intelligence needed to interpret and effectively utilize historical data, automating and enhancing compliance workflows as follows:
- Natural language understanding
Agentic AI uses a domain-trained LLM to interpret questions like “Show all trades in Q1 2023 where employees traded the same stocks as clients.” It extracts key entities (for example, employees, stocks, dates) and the intent (for example, insider trading detection).
- Reason agent for query planning
The Reason Agent maps the question to actual data sources, tables, and fields using a vectorized schema index. It plans joins, filters, and temporal constraints to retrieve the correct data, including bitemporal conditions such as “as of Q1.”
- Act agent for SQL generation
The Query Agent translates the plan into SQL or XTQL, optimized for XTDB’s bitemporal engine. It uses temporal predicates (for example, overlaps, precedes, during) to express time-bound conditions accurately. - Guardrails and validation
All queries pass through a Guard/Validator component that checks correctness, validates syntax against the database, enforces security policies, and ensures user permissions—delivering compliance-grade safety and explainability. - Execution and refinement
The query runs against XTDB, fetching results formatted into reports or charts. Agentic AI operates in a reasoning-action loop. If results seem off (for example, empty output despite expectations), the Reason Agent adjusts the query and the Query Agent regenerates a refined version. - Output in regulatory format
Final results can be exported as CSV, XML, or regulatory schemas such as FIX or ISO 20022. A UI allows compliance officers to review and approve responses before submission.
This agentic AI approach augments the compliance team rather than replacing them. It handles the heavy lifting of data retrieval and cross-referencing, while humans provide oversight and domain expertise.
How bitemporal data and agentic AI collaborate behind the scenes
Bringing these components together requires an architecture that integrates with a financial institution’s existing data landscape and ensures scalability and governance. This architecture can be viewed as a combination of data ingestion & storage and AI query & application layer, all underpinned by governance and security controls.
Market manipulation surveillance case study: Agentic AI regulatory compliance in action
One large exchange implemented this solution to investigate spoofing and layering in trading order books when a trader places orders they intend to cancel to mislead the market. Detection requires analyzing order entry and cancellation patterns with precise timing. Leveraging bitemporal data allowed the surveillance team to precisely replay the order book at any millisecond. AI agents automated queries to detect sequences like “5 or more rapid cancellations by Trader X followed by favorable price moves,” significantly reducing false positives and accelerating actionable evidence. Historical order states and AI-generated insights provided regulators with clear, audit-proof evidence, uncovering patterns previously too complex for manual detection.
Additional use cases including insider trading, best execution analysis, and trade allocation reviews benefit similarly, turning week-long investigations into efficient, regulator-ready outcomes within hours. Download the white paper to find out more.
Benefits and results
Adopting the Agentic AI and XTDB powered compliance solution yields significant benefits for financial institutions, translating into both quantitative gains and strategic advantages.
50%+ faster responses Respond to regulatory inquiries in hours instead of days, reducing response times by over 50%, helping you avoid penalties and improving your standing with regulators. | 30–40% reduced manual effort Automate data retrieval and analysis to slash manual effort by 30–40%, letting your compliance and IT teams focus on strategic initiatives instead of tedious tasks. | 100% audit-ready accuracy Bitemporal data provides accurate historical records with immutable audit trails, significantly reducing errors and boosting confidence in your compliance responses. | Scalable and future-proof Easily handle increasing volumes of complex inquiries and rapidly adapt to changing regulatory demands without extensive retooling. | Competitive advantage Proactively manage compliance risks, protect your reputation, and demonstrate superior governance, strengthening trust among regulators, investors, and clients. |
Ready to confidently respond to regulators—every time? Download our comprehensive white paper and discover how agentic AI and bitemporal data can help your business deliver faster, more accurate compliance responses, reduce operational costs, avoid fines, and enhance relationships with regulators.
Next time a regulatory request arrives, your compliance team can confidently say: “We have that information at our fingertips.”